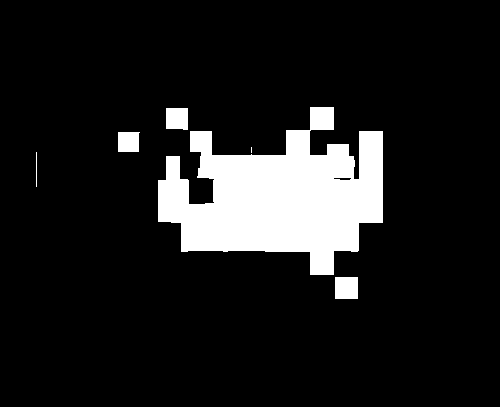
So the site is being updated – if you click on the ‘Neural Networks’ menu tab above and run through the sections you will see an example of an A.I. Perceptron running in real time on WordPress, no less – now it needs a little work and tidying up but, it works! So if you want to see a computer ‘learn’ then you know where to go – its right here. I will even tell you How it works too but, for now, I am still putting that bit together. Like I said, the Math was easy and so it is – until you write it down and it looks horrid so, I am trying to do the hard bit and get it to look simple….Look, if a dumb machine can do it then, so can you and I. Yes?
Image courtesy of : https://giphy.com
Описываемая публикация воодушевила меня и своими руками произвести что либо хорошее в пользу современных людей.
Thank you Лев – Lev. I am glad the article has your interest and hope you will find the rest of the subjects to come just as useful – Many greetings liz
Непревзойдённо. Убежден, этой информации можно довериться
Hi Vera and thanks yes, we try hard to make sure all the information is correct and as it is an open web site if we do make a mistake and some one tells us, then we will correct it quickly. Thanks
Извечно нужно не предавать забвению об, самом первостепенном,: любая информационная подборка должна нести полезность каждому. И конечно здесь это в целом имеются. Буду брать с Вас пример
Thanks Tatyana -I agree – Like I said to Вера – All information should be freely available to everyone and we try to do this by keeping the website open to all who wish to read it.
Спасибо за такую информацию
Thank you Marina – you are most welcome.
Спасибо большое вам за информацию
Hi Anna, many thanks I an glad you are happy with the information – keep reading as there is much more coming soon thank you liz.
One can only wonder by seeing art exhibitions if these deep dream states are visceral and lower layered in our brain. It is like the artists can connect to these abstract layers of cognition and create output that looks like dream states of contemporary neural networks. We are going to be able to simulate lots of psychiatric conditions in the near future by gaining insights into our cognition through the development of artificial neural networks. Future looks amazing to me for psychiatrists.
Hi Irena, many thanks for your thoughts – I agree with you. I have seen a video taken of all the Galaxies in space. The video takes you a fly though of all the filaments of the galaxy structures and many have said that it looks like you are drifting through the neural pathways of the mind. I have considered posting the video on the site as it is quite amazing but, I need to get permission from the owners first. Soon as I do I will post it here. Many thanks Liz
that spurious states are entir ely suppresse d with a prop er rest, allowing the network to achieve perfect Figure 5. Critical lines for ergodicity breaking (dotted curves) and retrieval region boundary (solid curves)
Many thanks Руслана – The article you are referring to is from Researchgate – by Elena Agliari et al. Where the researchers allowed a Neural Network time for learning, then allowed the network time to rest or sleep and possibly dream in order to assimilate the awakened learning phase. Most Interesting article that you can all read if you Click Here to go and look at the Researchgate site – The network in this case really is Dreaming in Binary – thanks again.
One challenge of using artificial neural networks is that it is near impossible to understand exactly what goes on inside of such a network. To this end, people at Google devised a way to probe the inner workings of an artificial neural network that they call DeepDream. DeepDream is most relevant for programs that recognize structure in images, often using a type of artificial neural network known as a deep convolutional neural network. The idea is to relieve tension between what the AI is given as input and what it might want to receive as input. That is, an image is distorted slightly to one that would better match the AI’s original interpretation of the image. While this sounds innocent enough, it can lead to some pretty bizarre images. This is mainly because an artificial neural network can often function well enough without complete confidence in its own answers or even really knowing what it is looking for in the image that is given. Real images always look at least somewhat strange or ambiguous to an AI, and distorting the image to forcibly reduce uncertainty from the AI’s point of view causes it to look strange to us. The images produced by DeepDream are a way of probing the uncertainty or tension in an artificial neural network, which is otherwise hidden (especially when the artificial neural network can only give binary yes or no answers.) DeepDream applied to a picture of the sky. Here, the neural network is trained to recognize locations, and is most familiar with furniture and buildings. It has never seen the sky before, and so initially it tries to make sense of it in terms of familiar shapes. DeepDream does the rest.
Many thanks laminate Kiev – you have made a very good comment. I cannot relly improve on your comment other than to say if anyone wishes to read more about Deep Dream then you can visit their website by Clicking Here : Deep Dream It is a very interesting site and if you look at 3 blue 1 brown on youtube he uses a similar technique to delve into the deeper areas of image processing in neural nets. Many thanks Liz.
In modern face recognition, the conventional pipeline consists of four stages: detect = align = represent = classify. We revisit both the alignment step and the representation step by employing explicit 3D face modeling in order to apply a piecewise affine transformation, and derive a face representation from a nine-layer deep neural network. This deep network involves more than 120 million parameters using several locally connected layers without weight sharing, rather than the standard convolutional layers. Thus we trained it on the largest facial dataset to-date, an identity labeled dataset of four million facial images belonging to more than 4,000 identities, where each identity has an average of over a thousand samples. The learned representations coupling the accurate model-based alignment with the large facial database generalize remarkably well to faces in unconstrained environments, even with a simple classifier. Our method reaches an accuracy of 97.25% on the Labeled Faces in the Wild (LFW) dataset, reducing the error of the current state of the art by more than 25%, closely approaching human-level performance.
Hello bulldozer Here you are referring to an article related to Deep Face – this is Facebooks AI system that looks at images and in your own images tries to interpret how you are feeling.(amongst other things) If you would like so see another opinion of deep face here is a small article by Hannah Carr you can see it by Clicking Here. Many thanks Liz.
Experience replay was introduced in 1991 by then Carnegie-Mellon Ph.D. student Long-ji Lin. It is a way of helping AI to learn tasks in which meaningful feedback either comes rarely or with a significant cost. The AI is programmed to reflect on sequences of past experiences in order to reinforce any possibly significant impressions those events may make on its behavior. In its original form, experience replay can be viewed as an ‘unregulated’ policy of encouraging an AI to approach nearby ‘feasible’ solutions and reject poor behaviors more rapidly. The idea is that significant events will naturally reinforce each other to make large impressions on the network. Meanwhile, the impressions of individual incoherent events will tend to cancel out. As long as the replay memory of the neural network is large enough, experiences of arbitrarily high significance can make appropriately large impressions on the state of the neural network.
Hi Progetto You are here referring to the piece by Henry Wilkin where he describes the dreaming structures in the ‘mind’ of A.I. It is a very well written and interesting article that is well worth a read. The paper is on the Harvard University web site and can be read by Clicking Here. Many Thanks Liz.