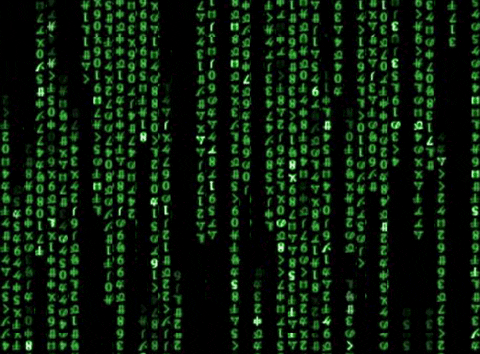
I created this site to explore the vast potential that Machine Intelligence could offer – indeed by reading through this site you will learn how Machines learn – knowledge is power. As of today, the internet has been around in popular culture for a generation or so – indeed there are some amongst us who have had the benefit of the internet throughout the whole of their lives and know of nothing else. I had a message today from a young relative asking if there was an ‘App’ that she could get which could list out a weeks worth of meal choices and give the list of ingredients to make the meal: Making meal choices and cooking a less of a chore. I thought – maybe a good idea but …. Maybe too much reliance on Machine Logic may, incapacitate our ability to think and choose for ourselves. Knowledge is still power.
Matrix image courtesy of : https://giphy.com/
“Абсолютно всё трудное состоит из простого.” Безумно хорошо, что Вы умеете донести информационную подборку не сложным, возможным для осмысления, способом.
Thank you Дмитрий – Please allow me to translate your message – Dmitry says – “Absolutely everything that is difficult consists of the simple. ” It is good that you are able to convey informational set in an easy way, possible for comprehension.” Thank you Dmitry. I hope that you are finding it enjoyable to read too. Liz
“Жизни верь, она ведь обучит эффективнее любых книг”. Пребольшое спасибо только за то, что вообще даёте данные, какая приходить на выручку в нашей жизни.
Thank you Олеся -Please allow a small translation – Olesya says ““Believe in life, it will teach more effectively than any books.” Thank you very much just for the fact that you give data on what kind of help is ( available ) in our life.” — This is all I am trying to do – I give information so that you can decide for yourself whether Machine Learning is a good thing or possibly not so good. I will be posting some more videos showing these choices as I go along. Many thanks – Liz
Жизнь человека никак не состоит в том, чтобы жить, а в оном, чтобы ощущать, что живешь. По завершении чтения Ваших размещённых статей, я, по подлинному, начинаю уразумевать, что у меня открывается смысл в жизни.
Hi Ivan – please allow a small translation as your comments are very interesting -“Human life does not in any way consist in living, but in it, in order to feel that you are living. Upon completion of reading your posted articles, I, in truth, begin to understand that I have a meaning in life.” – interesting but. apart from my articles – what is also – your meaning in life ?
Только лишь тот человек заслуживает жизни и независимости, кто ежедневно за них идет на бой. Я восхищаюсь, прочитывая Ваши тексты. Именно они дают мне глоток свободы в предстоящем дне.
Hi Hope – let me again translate – “Only that person deserves life and independence who goes to battle for them every day. I admire reading your texts. They are the ones who give me a breath of freedom in the day ahead.” Thank you Hope – A nice stream of thought. thank you Liz
Мне порекомендовал Ваш вебсайт отец одногрупницы моего сына. Хочу возблагодарить за столь нужную информационную подборку. Решил начиная с сегодняшнего дня подписаться на анонсы Вашего ресурса.
Hi Alina – please let me translate – “The father of my son’s classmate recommended your website to me. I would like to thank you for the much needed information collection. I decided to subscribe to the announcements of your resource starting today.” That is so nice of you to say so. I hope the information is of good to you.
Humans don’t start their thinking from scratch every second. As you read this essay, you understand each word based on your understanding of previous words. You don’t throw everything away and start thinking from scratch again. Your thoughts have persistence. Traditional neural networks can’t do this, and it seems like a major shortcoming. For example, imagine you want to classify what kind of event is happening at every point in a movie. It’s unclear how a traditional neural network could use its reasoning about previous events in the film to inform later ones.
Brilliant – this is the kind of thoughts that I am looking for – However to answer your question best as I can, machines learn from information they are given for a specific (and quite simple) task – to learn this they repeat the learning process over and over until the learning is completed. Once completed the machine no longer needs to learn from scratch but can use the information within its memory to do its task without any more learning being involved. Watching a movie as in your example would require an immense neural network because of the amount of information involved and as far as I know – there are no networks of this size and capacity yet. A machine may be able to recognise a few faces in the movie but as to understanding the story – as you quite rightly point out – that is far beyond what a current network could do. Many thanks liz.
Long Short Term Memory networks – usually just called “LSTMs” – are a special kind of RNN, capable of learning long-term dependencies. They were introduced by Hochreiter Schmidhuber (1997), and were refined and popularized by many people in following work. LSTMs are explicitly designed to avoid the long-term dependency problem. Remembering information for long periods of time is practically their default behavior, not something they struggle to learn!
Hi and many thanks for your comment – I agree, simple networks simply learn from scratch – starting all over again when new information is given. More complex networks need to be able to retain that information and add more information to it. This is where LSTM’s come in – this is a bit more of a subject than I can put into this brief post. However, there is a fine article by Colah on this subject which you can read by Clicking Here:- Colahs Blog LSTM. Many thanks Liz.
That is, suppose we wanted to write, say, a recognizer of handwritten numbers. If we were supersmart we could write an incredibly complex deterministic algorithm that we could use to classiy images into numbers. But since we are not that smart (in fact I m not sure an algorithm like that can even exist) we have to use a neural network. What I thought is that what the network was actually doing is 534 find the set of logic gates that most closely acts like the ideal algorithm (which is fundamentally also a set of logic gates).
Hello vavada I will be exploring the subject of handwriting recognition in my next posts – there is not a simple algorithm that can decipher handwriting however Neural Networks can. They do this by learning patterns ( similar to facial recognition ). These ideas are my next plan for topics on this site. Here the only logic used is:- Is this ‘pattern’ correct ? Yes – that is good so continue – or no – well guess again.( loop ) – Soon as I can organize it, it all it will be here, many thanks Liz.
Precisely what I was looking for, thanks for putting up. Stefa Horatius Cristina
Many thanks, we hope you have interest continuing as we go along.
In terms of media archaeology, the neural network invention can be described as the composition of four techno-logical forms: scansion (discretization or digitization of analog inputs), logic gate (that can be realized as potentiometer, valve, transistor, etc.), feedback loop (the basic idea of cybernetics), and network (inspired here by the arrangement of neurons and synapses). Nonetheless, the purpose of a neural network is to calculate a statistico-topological construct that is more complex than the disposition of such forms. The function of a neural network is to record similar input patterns (training dataset) as an of its nodes. Once an inner state has been calculated (i.e., the neural network has been ‘trained’ for the recognition of a specific pattern), this statistical construct can be installed in neural networks with identical structure and used to recognize patterns in new data.
Hi Krasnoyarsk I see you quote from an article by Matteo Pasquinelli. It is an interesting article which gives good background to A.I – if you wish to read the full article you can read it on Glass Bead if you Click Here.Many Thanks Liz
I must say I read a great article with pleasure
Thank you for your consideration -liz.
Very informative blog article.Much thanks again. Fantastic.
Many thanks necole – your website too is Fantastic — Many thanks Liz.
Conventional adaptive control techniques have, for the most part, been based on methods for linear or weakly non-linear systems. More recently, neural network and genetic algorithm controllers have started to be applied to complex, non-linear dynamic systems. The control of chaotic dynamic systems poses a series of especially challenging problems. In this paper, an adaptive control architecture using neural networks and genetic algorithms is applied to a complex, highly nonlinear, chaotic dynamic system: the adaptive attitude control problem (for a satellite), in the presence of large, external forces (which left to themselves led the system into a chaotic motion). In contrast to the OGY method, which uses small control adjustments to stabilize a chaotic system in an otherwise unstable but natural periodic orbit of the system, the neuro-genetic controller may use large control adjustments and proves capable of effectively attaining any specified system state, with no a prioriknowledge of the dynamics, even in the presence of significant noise.
Thank you Svetlana — you are referring to rather esoteric article by Dracopoulos et al on the Adaptive neuro-genetic control of chaos !! Referring to Satellites — Now, this is a little too esoteric for my little blog but anyone who wishes to read it can find it by Clicking Here I must admit that I have read many ‘Jargon Infested Bi Polar Anally Infused (A.I) Articles ‘ But this one really takes the Biscuit. It’s great, many thanks for finding this Gem – Liz – not only that I have read it and it kind of does make sense as long as you CAN possibly replace the Lyapunov function with this algorithm !!
Computational neuroscience differs in a crucial respect from CCTM and connectionism: it abandons multiply realizability. Computational neuroscientists cite specific neurophysiological properties and processes, so their models do not apply equally well to (say) a sufficiently different silicon-based creature. Thus, computational neuroscience sacrifices a key feature that originally attracted philosophers to CTM. Computational neuroscientists will respond that this sacrifice is worth the resultant insight into neurophysiological underpinnings. But many computationalists worry that, by focusing too much on neural underpinnings, we risk losing sight of the cognitive forest for the neuronal trees. Neurophysiological details are important, but don t we also need an additional abstract level of computational description that prescinds from such details? Gallistel and King (2009) argue that a myopic fixation upon what we currently know about the brain has led computational neuroscience to shortchange core cognitive phenomena such as navigation, spatial and temporal learning, and so on. Similarly, Edelman (2014) complains that the Neural Engineering Framework substitutes a blizzard of neurophysiological details for satisfying psychological explanations.
Hello Casino I see you are quoting from an excellent article taken from The Stanford Encyclopedia entitled “The Computational Theory of Mind.” CTM first published in 2015. It is a well researched article and well worth a read. It goes into a lot more history than I have been able to put into this little blog – So if anyone wishes to read the original you can find it by Clicking Here. Many Thanks for your diligent research Liz.
I’m truly enjoying the design and layout of your blog.
It’s a very easy on the eyes which makes it much more pleasant for me to come here and visit more often. Did you hire out
a developer to create your theme? Exceptional work!
Hello Traffic many thanks for your comment and, in answer to your question – I did not hire a developer for the theme it is all done by me, however I do get ‘help’ with some of the extra Machine Learning coding on the site. Many thanks Liz.